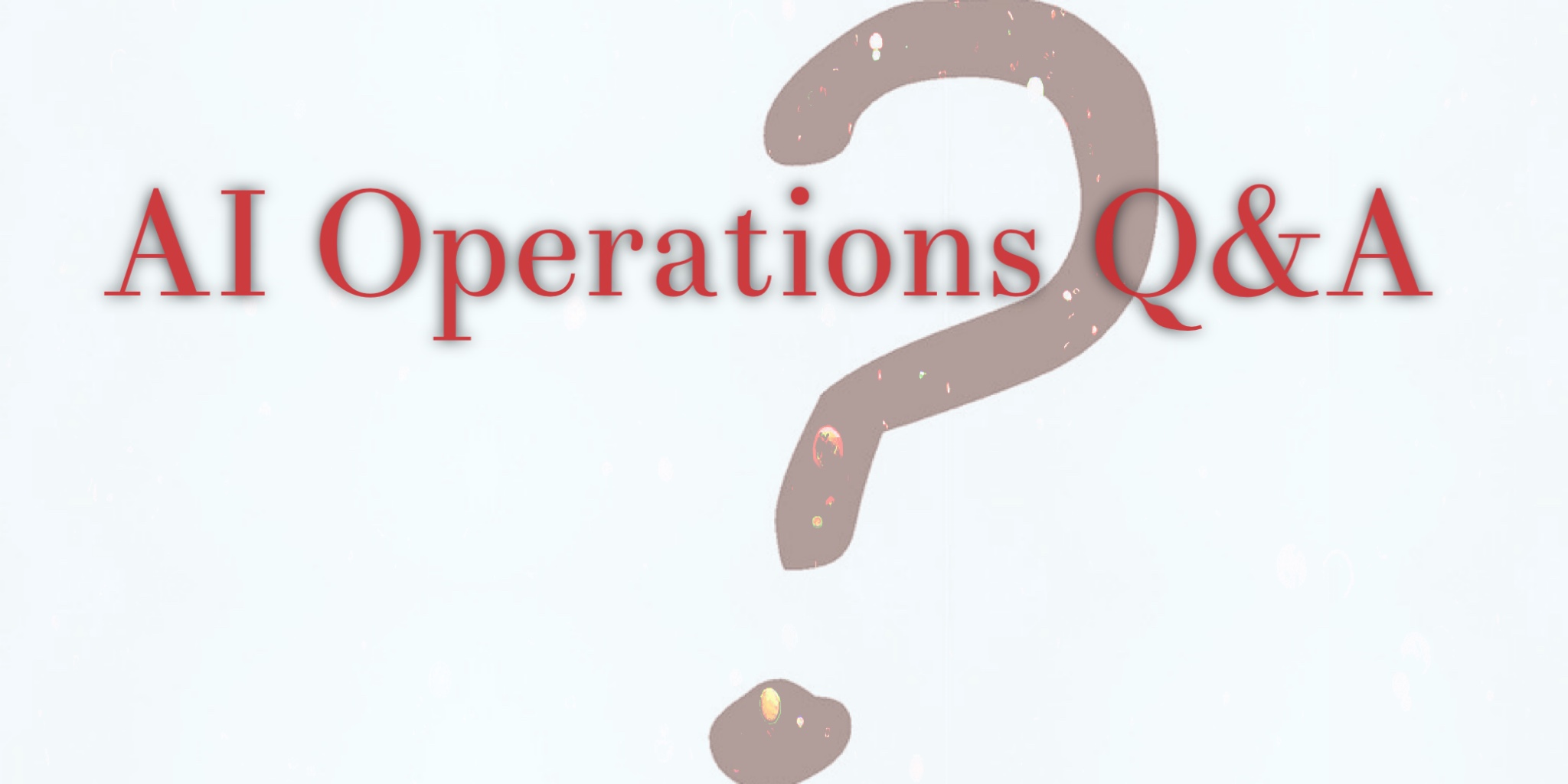
- Data migration only
- Re-platforming
- Re-architecting
- Re-factoring
Sep 14, 2020
Jul 23, 2020
Jun 15, 2020
It was April Fools’ Day, but the Artificial Intelligence (AI) webcast the SNIA Cloud Storage Technologies Initiative (CSTI) hosted on April 1st was no joke! We were fortunate to have AI experts, Glyn Bowden and James Myers, join us for an interesting discussion on the impact AI is having on data strategies. If you missed the live event, you can watch it here on-demand. The audience asked several great questions. Here are our experts’ answers:
Q. How does the performance requirement of the data change from its capture at the edge through to its use
A. That depends a lot on what purpose the data is being
captured for. For example, consider a video analytics solution to capture
real-time activities. The data transfer will need to be low latency to get the
frames to the inference engine as quickly as possible. However, there is less
of a need to protect that data, as if we lose a frame or two it’s not a major
issue. Resolution and image fidelity are already likely to have been sacrificed
through compression. Now think of financial trading transactions. It may be we
want to do some real-time work against them to detect fraud, or feedback into a
market prediction engine; however we may just want to push them into an archive.
In this case, as long as we can push the data through the acquisition function
quickly, we don’t want to cause issues for processing new incoming data and have
side effects like filling up of caches etc, so we don’t need to be too concerned with
performance. However, we MUST protect every transaction. This means that each
piece of data and its use will dictate what the performance, protection and any
other requirements are required as it passes through the pipeline.
Q. Need to think of the security, who is seeing the data resource?
A. Security
and governance is key to building a successful and flexible data pipeline. We
can no longer assume that data will only have one use, or that we know in
advance all personas who will access it; hence we won’t know in advance how to protect
the data. So, each step needs to consider how the data should be treated and
protected. The security model is one where the security profile of the data is
applied to the data itself and not any individual storage appliance that it
might pass through. This can be done with the use of metadata and signing to
ensure you know exactly how a particular data set, or even object, can and
should be treated. The upside to this is that you can also build very good data
dictionaries using this metadata, and make discoverability and audit of use
much simpler. And with that sort of metadata, the ability to couple data to
locations through standards such as the SNIA
Cloud Data Management Interface (CDMI) brings real opportunity.
Q.
Great overview on the inner workings of AI. Would a company’s Blockchain have a
role in the provisioning of AI?
A.
Blockchain can play a role in AI. There are vendors with patents around Blockchain’s
use in distributing training features so that others can leverage trained
weights and parameters for refining their own models without the need to have
access to the original data. Now, is blockchain a requirement for this to
happen? No, not at all. However, it can provide a method to assess the providence
of those parameters and ensure you’re not being duped into using polluted
weights.
Q.
It looks like everybody is talking about AI, but thinking about pattern
recognition / machine learning. The biggest differentiator for human
intelligence is – making a decision and acting on its own, without external
influence. Little children are good example. Can AI make decisions on its own
right now?
A.
Yes and no. Machine Learning (ML) today results in a prediction and a
probability of its accuracy. So that’s only one stage of the cognitive pipeline
that leads from observation, to assessment, to decision and ultimately action.
Basically, ML on its own provides the assessment and decision capability. We
then write additional components to translate that decision into actions. That
doesn’t need to be a “Switch / Case” or “If this then that”
situation. We can plug the outcomes directly into the decision engine so that the
ML algorithm is selecting the outcome desired directly. Our extra code just
tells it how to go about that. But today’s AI has a very narrow focus. It’s not
general intelligence that can assess entirely new features without training and
then infer from previous experience how it should interpret them. It is not yet
capable of deriving context from past experiences and applying them to new and
different experiences.
Q.
Shouldn’t there be a path for the live data (or some cleaned-up version or
output of the inference) to be fed back into the training data to evolve and
improve the training model?
A. Yes
there should be. Ideally you will capture in a couple of places. One would be
your live pipeline. If you are using something like Kafka to do the pipelining you
can split the data to two different locations and persist one in a data lake or
archive and process the other through your live inference pipeline. You might
also then want your inference results pushed out to the archive as well as this
could be a good source of “training data”; it’s essentially labelled
and ready to use. Of course, you would need to manually review this, as if
there is inaccuracy in the model, a few false positives can reinforce that
inaccuracy.
Q.
Can the next topic focus be on pipes and new options?
A. Great Idea. In fact, given the popularity of this presentation, we are looking at a couple more webcasts on AI. There’s a lot to cover! Follow us on Twitter @sniacloud_com for dates of future webcast.
Mar 13, 2020
Mar 13, 2020
Mar 1, 2018
Jan 29, 2018
Leave a Reply